Creativity is one of the most complex areas to explore and define in the evolving field of artificial intelligence. pease and colton 2011 a influential work in 2011 introduced a new framework for computational creativity that aimed to establish a structured approach to understanding how creativity could be generated, evaluated, and even improved through AI systems. Their contributions provided insights into how machines could go beyond mere automation to produce original, valuable work that resonates with human concepts of creativity.
This article delves into the key concepts, methodology, and implications of Pease and Colton’s 2011 study on computational creativity, focusing on how their framework has impacted both the development of creative AI and the broader understanding of creativity in human contexts.
Defining Computational Creativity

Computational creativity enables machines to perform tasks typically associated with human creativity, such as art, music, literature, and problem-solving. The goal is not simply replicating existing work but enabling AI systems to create something novel and meaningful. Pease and Colton’s approach emphasized the importance of machines being able to generate new ideas, evaluate them, and refine their creative process over time, a concept known as “iterative creativity.”
In traditional terms, creativity often relies on subjective judgment. pease and colton 2011 a framework aimed to formalize this by introducing criteria and processes that could be objectively measured and replicated in computational systems. Their research suggested that with suitable algorithms and data inputs, machines could produce outcomes that meet accepted standards of creativity.
Critical Concepts in Pease and Colton’s Framework
Pease and Colton (2011) identified several crucial components of creativity that could be analyzed and engineered in AI systems:

- Novelty: For creative output to be precious, it must offer something new and original. pease and colton 2011 a examined how machines could be programmed to avoid repetition and instead produce fresh and unpredictable outcomes.
- Quality and Value: Creativity is not only about producing something new; it must also have quality and meaning. The framework they developed included methods for assessing the practical value and quality of AI-generated work.
- Intentionality and Process: Human creativity often involves intentional actions and understanding the creative process. Pease and Colton’s framework incorporated elements that allowed AI to simulate decision-making processes, thus giving the output an appearance of intentional design.
- Adaptability and Learning: Pease and Colton emphasized that interaction and feedback should improve a system’s creative abilities over time. This adaptability ensures that AI does not stagnate in its creative capacity but continues evolving based on prior outputs and learned experiences.
Methodology of the Pease and Colton (2011) Study
The methodology pease and colton 2011 a used in their 2011 study was structured around implementing and testing their framework in real-world scenarios. This was achieved through visual art and narrative creation experiments, where the outcomes could be readily analyzed and evaluated for creativity.
Their methodology involved creating algorithms that could simulate various stages of the creative process, from idea generation to refinement and evaluation. Pease and Colton designed a series of tests to measure the novelty and quality of the output, ensuring that the results met predefined standards of creativity. This approach allowed them to assess whether humaAI’s servers could perceive the AI’s output as genuinely creative.
Impact on the Field of Artificial Creativity
Pease significantly influenced computational creativity, paving the way for further research into how machines can engage in creative processes that were once considered uniquely human. Their framework has provided a foundation for advancements in creative AI, particularly in generative art, music composition, and story creation.
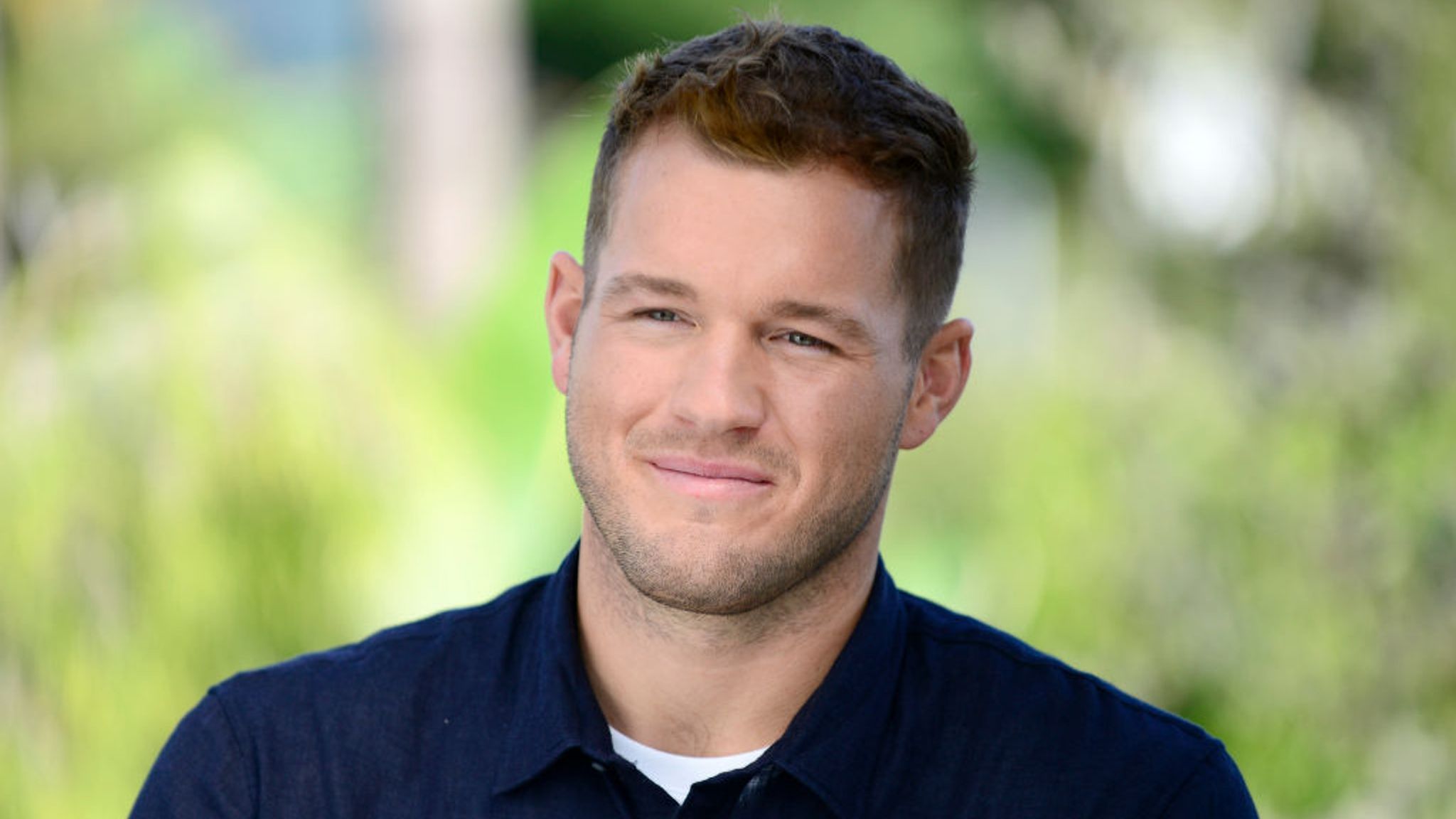
The implications of their research have also influenced practical applications in industries ranging from entertainment to marketing, where AI-driven creativity has proven valuable in generating unique and engaging content and by establishing methods to evaluate and improve machine creativity, pease and colton 2011 a helped to bridge the gap between automation and authentic creative expression in AI systems.
Challenges and Critiques of Computational Creativity
While Pease and Colton’Colton’sork were groundbreaking, it also faced critiques and challenges, particularly regarding the subjective nature of creativity. Many scholars argue that creativity cannot be fully quantified or reduced to algorithms, as it often involves complex emotions, cultural influences, and unique personal experiences that AI may struggle to replicate.
Another challenge lies in evaluating machine creativity. Human observers may have biases or limitations in recognizing creativity in non-human outputs. pease and colton 2011 a acknowledged these challenges, suggesting that future research must address the inherent subjectivity in creativity evaluations and explore ways to make computational creativity more universally acceptable.
The Ethical and Philosophical Implications
The ability of machines to generate creative output raises important ethical and philosophical questions. pease and colton 2011 a Colton’sork encourage discussions around the authorship and ownership of machine-generated work and the potential impact of AI creativity on human jobs and culture. If machines can create art, write stories, and solve problems creatively, what implications does this have for human identity and the value of human-created work?
Some argue that machine creativity could dilute human culture, as the line between human and machine-generated content becomes increasingly blurred. Others believe that AI creativity could complement human efforts, offering new perspectives and possibilities for collaboration between humans and machines.
Applications and Future Directions
Pease and Colton’Colton’sas inspired ongoing research into how computational creativity can be applied across various fields. In education, for instance, creative AI tools are used to enhance learning experiences, helping students engage in creative problem-solving and exploration. In the business world, creative AI is generatetoageneratentent, product design cu productsstomer servfacilitate ice interactions.
Future research based on pease and colton 2011 a Colton’sork may involve refining algorithms to produce more nuanced and culturally aware creative outputs. As AI systems access more complex data and processing capabilities, they may be able to simulate deeper levels of understanding and creativity, potentially producing work indistinguishable from human-created content.
Conclusion: The Lasting Influence of Pease and Colton’Colton’sork
Pease and Colton’Colton’study remains a pivotal contribution to computational creativity. By proposing a framework for understanding and implementing machine creativity, they opened new avenues for exploring what it means to be creative and how machines might participate in creative processes. While challenges remain in defining and evaluating AI creativity, Pease and Colton’Colton’sas laid the groundwork for future advancements, providing a roadmap for AI researchers to follow.
As technology continues to evolve, pease and colton 2011 a’sts will remain relevant. They will push the boundaries of creativity and allow AI to contribute meaningfully to human culture and knowledge. The questions raised by their framework will likely guide discussions on the role of AI in society for years to come as we continue to explore the vast and uncharted territory of machine-generated creativity.
Leave a Reply